Smooth Contextual Bandits: Bridging the Parametric and Nonparametric Regret Regimes
Nathan Kallus – Cornell University
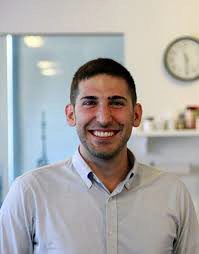
Abstract
Stochastic contextual bandits model dynamic, individualized decision-making when one must balance exploration and exploitation, with applications ranging from healthcare to e-commerce. The key objects are the regression functions of reward of an arm given context, and while it makes intuitive sense that the harder it is to learn these functions, the more exploration is necessary and the higher regret we should expect, the precise relationship is unknown. To study this we consider a nonparametric stochastic contextual bandit problem where the reward regressions are assumed to have Hölder smoothness parameter β (roughly, the number of derivatives). We develop a novel algorithm that carefully adjusts to all smoothness settings and we prove its regret is minimax rate-optimal by establishing matching upper and lower bounds. We show how this bridges a gap between two extremes that were previously studied in isolation: non-differentiable bandits (β≤1), where rate-optimal regret is achieved by running separate non-contextual bandits in different context regions, and parametric-response bandits (β=∞), where rate-optimal regret can be achieved with minimal or no exploration due to infinite extrapolatability. While our new algorithm bridges between such algorithmic extremes that exclusively use global or local information, our minimax regret results precisely characterize the continuous relationship between achievable regret and the complexity of learning.