Estimating Inflection Points in Community-level Homeless Rates with a Bayesian Nonparametric Mixture Model
Christopher Glynn – University of New Hampshire
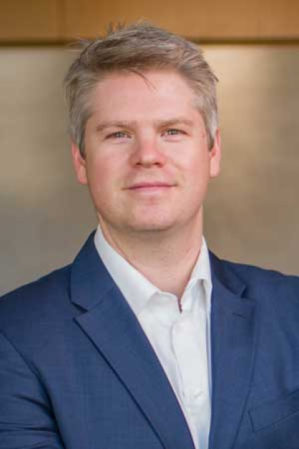
Abstract
Statistical models of community-level homeless rates typically assume a linear relationship to covariates. This linear model assumption precludes the possibility of inflection points in homeless rates – thresholds in quantifiable metrics of a community that, once breached, are associated with large increases in homelessness. In this talk, we model homeless rates as a flexible surface over measures of housing affordability and extreme poverty. We utilize the Ewens-Pitman attraction distribution to develop a Bayesian nonparametric mixture model in which clusters of communities with similar covariates share common patterns of variation in homeless rates.
A main finding of the study is that the expected homeless rate in a community increases sharply once median rental costs exceed 32% of median income, providing a statistical link between homelessness and the widely used definition of a housing cost burden at 30% of income. Our analysis also identifies clusters of communities that exhibit distinct geographic patterns, yielding insight into the homelessness and housing affordability crisis unfolding on both coasts of the United States.